Project Name
BiasedBeans
Role
UI/UX Designer, UX Researcher, Project Lead
Team: Jei Park (Solo)
Duration
Fall 2024 (12 weeks)
Objective
Algorithmic biases are not abstract technical issues as they
have real-world consequences that perpetuate systemic inequities
and harm marginalized communities.
In this project, I aim to design an engaging and user-centric platform
that empowers individuals to identify, report, and address generative
AI biases, fostering transparency, accountability, and meaningful social
impact.
Problem
Users often feel discouraged from actively reporting generative AI biases due to a lack of transparency, clear incentives, and a meaningful connection to real-world impact.
Reporting AI biases is deeply personal as it is driven by the same emotions they feel when confronting broader societal issues like gender inequality or unequal access to education. However, current reporting platforms fail to leverage this emotional connection, providing no clear pathway from bias reporting to tangible social impact.
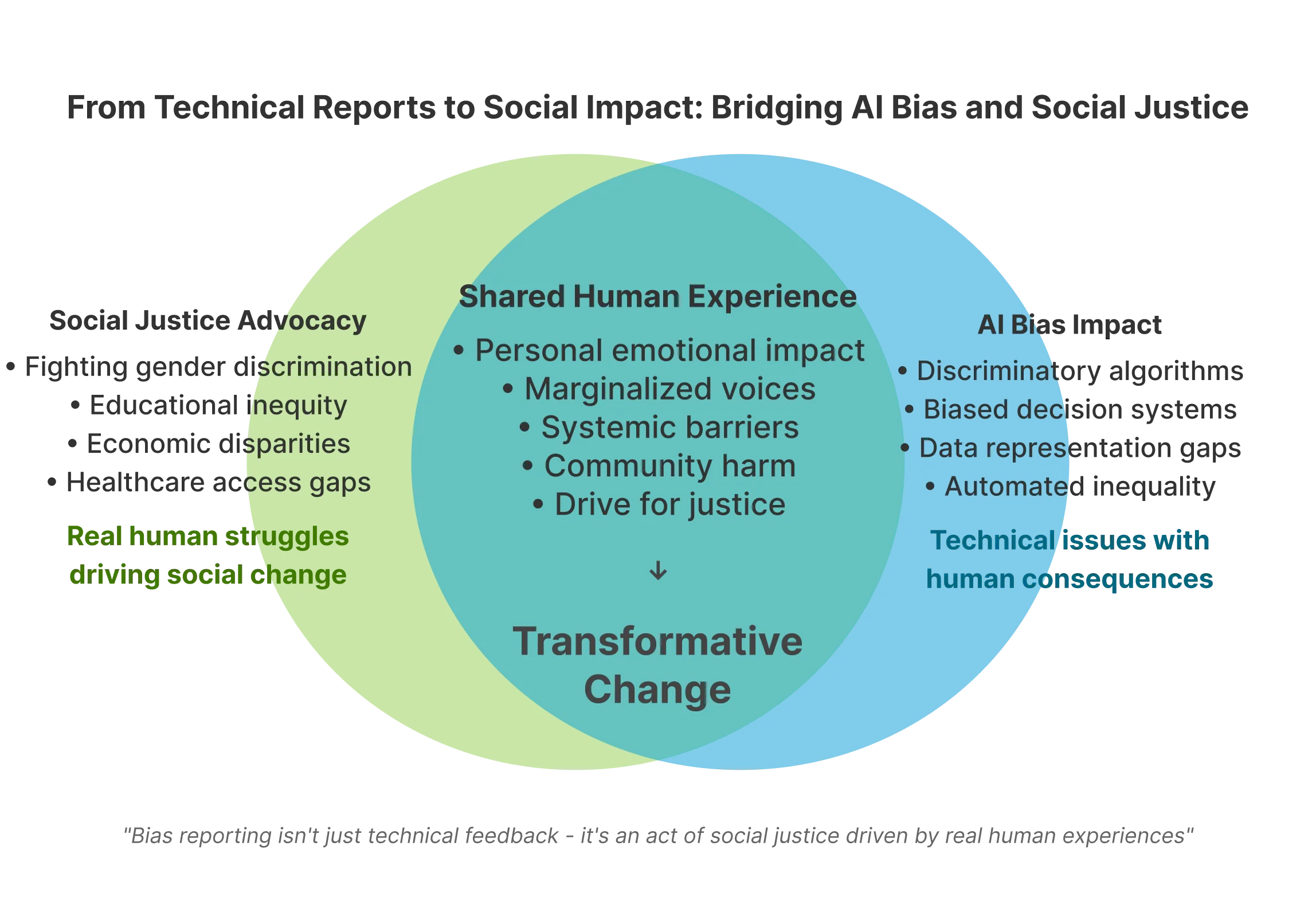
Needs
"I want to see how my efforts to report AI biases actually help make a difference, so I feel like I'm contributing to something meaningful."
"I want a simple and intuitive platform that helps me identify biases and makes reporting them easy and rewarding."
"I want to support causes I care about and feel like my actions have a real-world impact."
Opportunities Gap
There is an opportunity to create a platform that transforms AI bias reporting into a meaningful, empowering experience by connecting users' emotional motivation to tangible social impact.
There we can create a self-reinforcing cycle where users feel valued, see their contributions driving change, and are motivated to continue engaging.
How Might We
"How might we design an engaging and rewarding platform that motivate users identify and report generative AI biases, while helping them feel their contributions make a meaningful impact?"
Methods
We adopted a human-centered design approach to deeply
understand user needs and rapidly iterate on prototypes.
Through a blend of qualitative research, empathy-driven synthesis, and iterative testing, we gathered insights, identified key pain points, and refined our concepts.
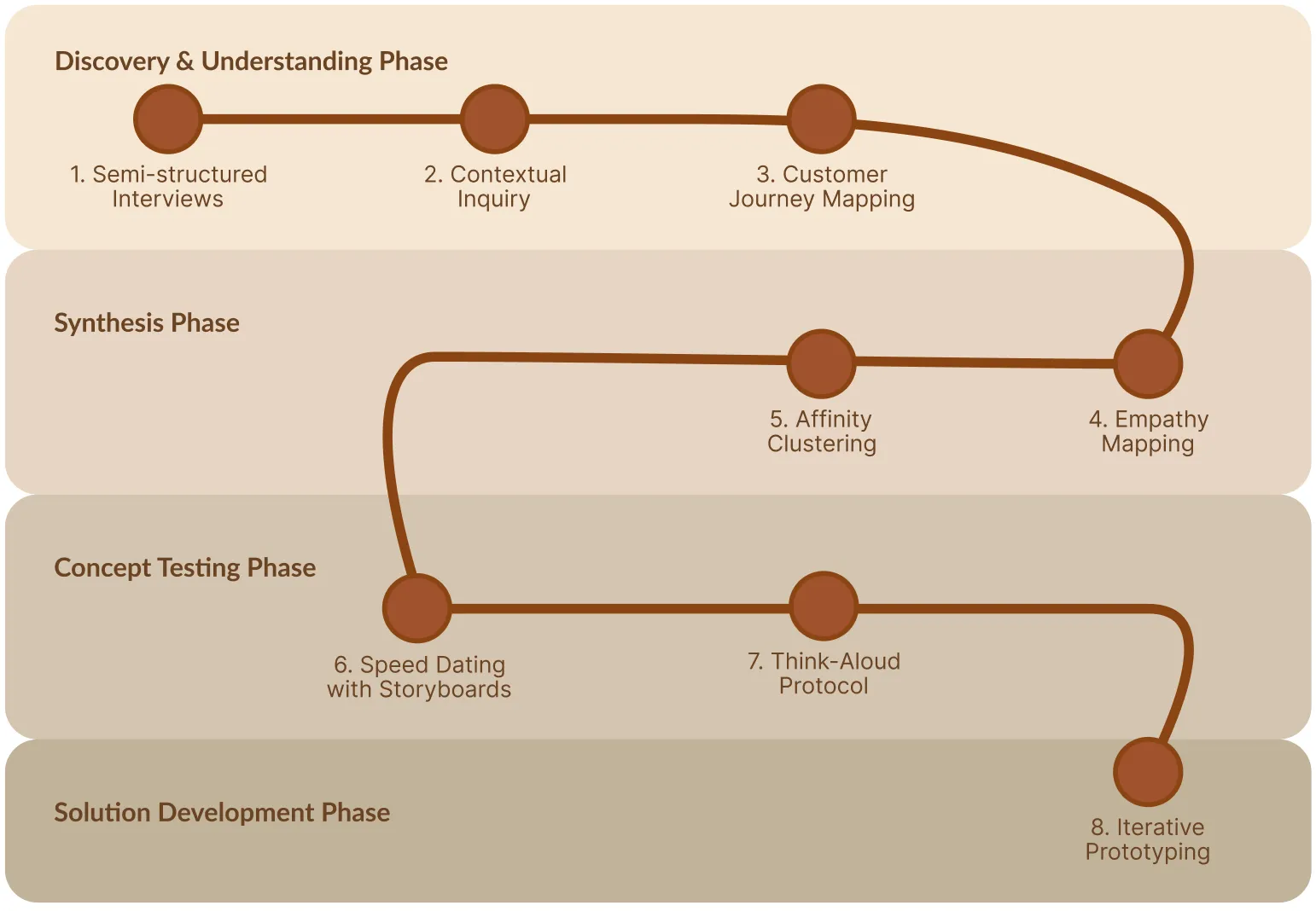